We are a multidisciplinary research team comprising of computer scientists, civil engineers, social scientists, urban planners, and public transportation experts dedicated to designing innovative solutions for enhancing public transportation operations. Our focus is on improving availability, reliability, effectiveness, and efficiency. Funded through federal grants and in collaboration with partner agencies such as the Chattanooga Area Regional Transportation Authority (CARTA) and Nashville WeGo, we employ cutting-edge AI approaches to address integrated multi-modal logistics challenges at scale, incorporating both same-day and long-term future trends. A key aspect of our work is the design of models for real-time energy consumption of mixed-vehicle fleets, including electric, hybrid, and diesel vehicles. These models enable us to predict and optimize operations to reduce overall energy impact while maintaining system-wide capacity. The team includes members from the Institute of Software Integrated Systems at Vanderbilt University, Cornell University, Pennsylvania State University, University of Washington, University of Tennessee at Chattanooga, University of South Carolina, Chattanooga Area Regional Transit Authority and Siemens Corporate Technology. This team consists of people with complementary backgrounds in transit operations, transit optimization, simulations, cyber-physical system, distributed system and software design and artificial intelligence. They have extensive prior experience in building transit and congestion performance indicators using machine-learning models that incorporate exogenous factors, such as weather, traffic, and public events. The research efforts that led to these projects were motivated by the White House’s smart cities initiative and the first Global Cities Team Challenge. The project was started by a collaboration between the Smart and Resilient Computing for Physical Environments Lab (SCOPE), WeGo Nashville and Chattanooga Area Regional Transit Authority. The funding for the investigative works performed by this alliance has been provided by National Science Foundation, Department of Energy and Federal Transit Authority.
R&D Spotlights
Microtransit and Paratransit Pilot Operations
The SmartTransit operations system was recently tested in the Clifton Hills area of Chattanooga. The pilot was conducted over a span of 27 service days between June and July 2024. On these service days, a vehicle, driver, and booking agent were deployed between 9 am and 3 pm. The insights gained from this pilot will be instrumental in refining and expanding microtransit services to better serve the community. Our long-term vision is to provide on-demand microtransit services that act as feeders for high-capacity, fixed-route transit services. Implementing this vision will enable agencies to provide energy-efficient and equitable transit access in areas with low population density, which are often underserved by existing transit solutions, by combining the energy efficiency of high-capacity transit with the flexibility of microtransit. The Clifton Hills microtransit pilot served to demonstrate and evaluate our novel technology solutions for on-demand transit. Previously our team tested the system for the CARTA paratransit operations and saw major improvements. Here are the key publications describing the deployment and pilot operations - IJCAI 2024 Demo, Paratransit Pilot
MicroTransit and ParaTransit Operations Software
The SmartTransit operations system is a modular on-demand public transportation routing system designed to enhance microtransit and paratransit services. It integrates advanced vehicle routing algorithms into the daily operations of transit agencies, addressing the challenges posed by varying objectives and constraints. This system includes management software for dispatchers and mobile applications for drivers and users. The software has been validated and demonstrated in a southern USA city, in separate microtransit and paratransit pilots, showing significant improvements in operational efficiency, energy efficiency, and cost-effectiveness in both cases. Here are the key publications describing the vehicle routing algorithms related to this work - Offline Routing With Negotiations @IJCAI 2022, Non-Myopic Online Routing @ICCPS 2022 Offline Scalable Routing With Rolling Horizons @AAAI 2023
Fixed Line Operations with WeGo
Public transit systems provide critical services for large sections of modern communities. Thus, on-time performance and reliable quality of service is important in maintaining ridership. However, disruptions in the form of overcrowding, vehicular failure, and accidents often lead to a degradation in service performance. Current approaches rely heavily on domain expertise by transit agency operators, often resulting in static dispatch locations. We develop AI systems aimed at improving public transit operations by optimizing the stationing and dispatch of substitute buses via data driven models. We also developed Vectura, a dashboard for Nashville’s public transportation network. This provides visualization tools to supplement transit operators by providing an information-rich portal for monitoring bus headway and ridership. - ICCPS 2024 (Best Paper Award), AAMAS 2024
Publication Spotlight
Deploying Mobility-On-Demand for All by Optimizing Paratransit Services was accepted at IJCAI 2024.
An Online Approach to Solving Public Transit Stationing and Dispatch Problem was awarded the best paper at ICCPS 2024.
Forecasting and Mitigating Disruptions in Public Bus Transit Services was presented at AAMAS 2024.
Focus Areas
Energy Efficiency
We are developing models to analyze and optimize the cost of transit operations by focusing on the energy impact of the vehicles. For this purpose, we are developing real-time data sets containing information about engine telemetry, including engine speed, GPS position, fuel usage, and state of charge (electrical vehicles) from all vehicles in addition to traffic congestion, current events in the city, and the braking and acceleration patterns. These high-dimensional datasets allow us to train accurate data-driven predictors using deep neural networks, for energy consumption given various routes and schedules. Having these predictors combined with traffic congestion information obtained from external sources will enable the agencies to identify and mitigate energy efficiency bottlenecks within each specific mode of operation such as electric bus and electric car. To make this possible, the project is also developing new distributed computing and machine learning algorithms that can handle data at such a rate and scale.
Microtransit Operations
We are developing microtransit dispatch algorithms that can serve passengers using dynamically generated routes and may expect passengers to make their way to and from common pick-up or drop-off points. Our hypothesis in the project is that the integration of data-driven methods with better operational research methods combined with a socially engaged design will lead to success. A key aspect of this research area is the development of techniques to preserve privacy across multimodal datasets, while also providing sufficient information for analysis and scheduling. This approach is different from commercial alternatives, because most commercial alternatives emphasize only on economic objectives, and as such, those services are often not integrated with public transit and do not address equity issues, which is a critical concern for us. The outcome of the project will be a deployment-ready software system that can be used to design and operate a micro-transit service effectively.
Fixed Line and Paratransit Operations
Lastly, we are developing algorithms to perform system-wide optimization, (the microtransit, fixed line and paratransit) focusing on three objectives: minimizing energy per passenger per mile, minimizing total energy consumed, and maximizing the percentage of daily trips served by public transit. While it is possible to optimize these decisions separately as prior work has done, integrated optimization can lead to significantly better service (e.g., synchronizing flexible courtesy stops with microtransit dispatch for easy transfer). However, this is hard due to uncertainty of future demand, traffic conditions etc. We address these challenges using state-of-the-art artificial intelligence, machine learning, and data-driven optimization techniques. Deep reinforcement learning (DRL) and Monte-Carlo tree search form the core of our operational optimization, which is supported by data-driven optimization for offline planning and by machine learning techniques for predicting demand, maintenance requirements, and traffic conditions.
People
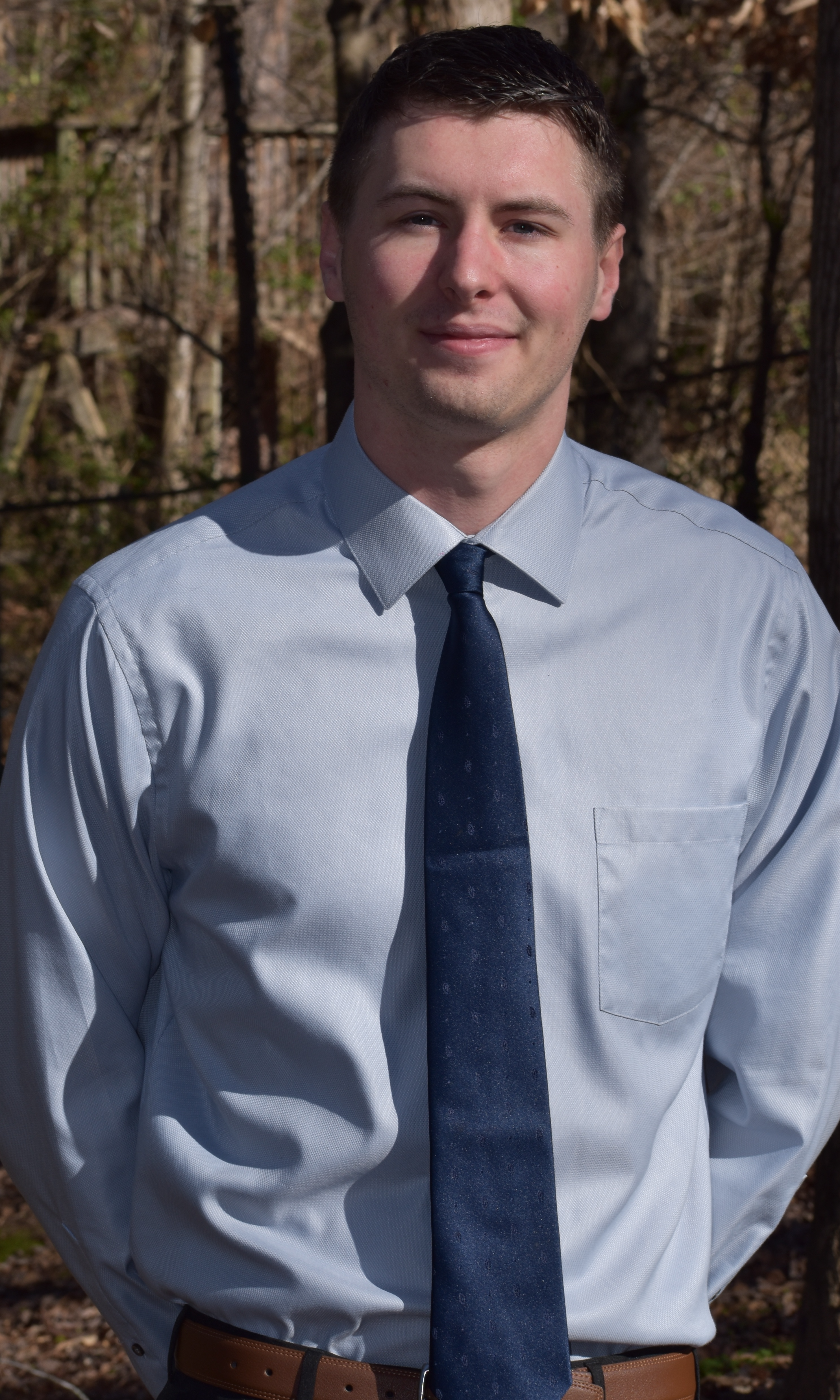
David Rogers
Research Engineer
Vanderbilt University
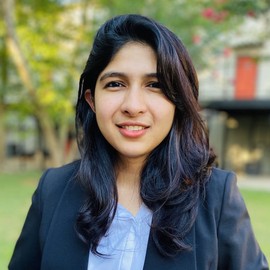
Afiya Ayman
Graduate Research Assistant
University of Houston